Articles
Why traditional RFM models aren’t enough for marketers today
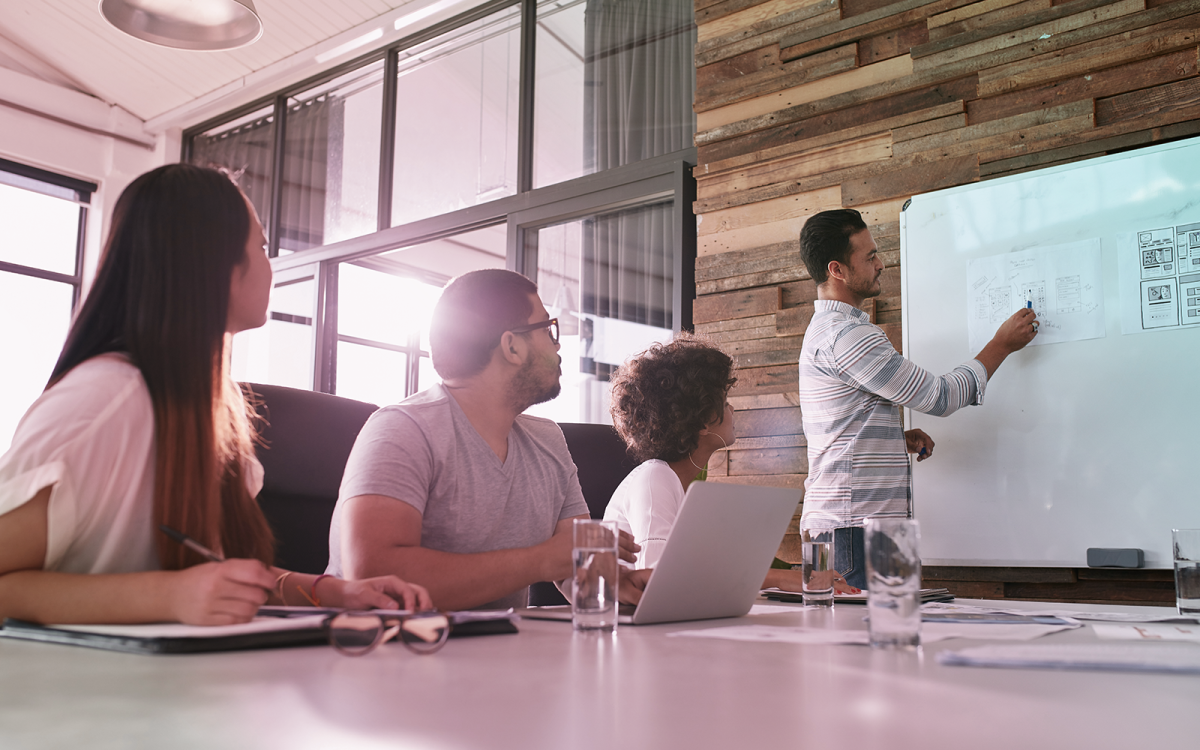
RFM models are a great tool relied on by marketing teams to segment and engage customers based on three factors – recency, frequency, and monetary value.
Insight from RFM analysis enables marketers to increase revenue by targeting specific groups or cohorts of customers with communications and offers that are more likely to be relevant based on data about a particular set of behaviours. This leads to increased response rates, customer retention, customer satisfaction, and customer lifetime value.
How Does RFM Analysis Work?
Market research has traditionally concentrated on high-level (not at customer-level granularity) demographic and psychographic information sourced from third-party surveys, which marketers try to use to conduct customer segmentation. Those data points are then applied to predict customer behaviour across much larger populations that share the same set of traits.
Traditional methods to develop RFM analyses were dependent on manual work using spreadsheets or other analysis tools, which in many cases could not handle a vast customer population. This led to many RFM models being produced using small data samples, hoping that they would be representative of the entire population, and/or establishing rules-of-thumb on common belief from managers and marketers on what recency, frequency and monetisation could mean.
For example, are “new” customers those who bought in the last 30 days or 60 days? These rules, extracted from either small customer samples or directly imposed using non-tested empirical knowledge, could lead to wrong assumptions, which can quickly snowball since they are then used across the business, leading teams to build products and insights over them.
With the advent of systems like customer data platforms that help gather, unify and synthesise customer behaviours, marketers have much more granular data about the habits of individual customers to inform segmentation. Rather than segmenting customers only using demographic and psychographic statistics per cohort or area of study, marketers can create segments based on the real-world behaviour of their actual customers, including purchase history, their attraction to discounts, coupons, vouchers and/or bundles, their satisfaction and engagement levels, reasons that led them to return items in the past, and all of this across any channel (online or offline), browsing history, prior campaign responses and more. Unsurprisingly, this type of segmentation is called behavioural segmentation.
Yet, even entry-level CRM system can perform rudimentary tracking of the three easily quantifiable characteristics that contribute to RFM analysis:
- Recency: This refers to the amount of time since a customer’s last interaction with a brand, which can include their last purchase, a visit to a website, use of a mobile app, a “like” on social media and more. Recency is a key metric because customers who have interacted with your brand more recently are more likely to respond to new marketing efforts.
- Frequency: This refers to the number of times a customer has made a purchase or otherwise interacted with your brand during a particular period of time. Frequency is a key metric because it shows how deeply a customer is engaged with your brand. Greater frequency indicates a higher degree of customer loyalty.
- Monetary: This refers to the total amount a customer has spent purchasing products and services over a particular period of time. Monetary value is a key metric because the customers who have spent the most in the past are more likely to spend more in the future.
Time for a New RFM Model
However, not all RFM models are equal. In fact, the RFM model used today by most marketers is no longer sufficient to effectively acquire, grow and retain customers. Here are five major issues facing marketers relying on traditional RFM models:
- Traditional RFM models are static and lack the fluidity required to adapt to changes in customer behaviour and preferences. A group of customers known to you last month may quickly change in a matter of days so when you send your communications and content, they will no longer be personalised and relevant.
- Traditional RFM models are built on historical data, and don’t factor in what your customers are doing right now in the moment, across physical and digital channels. Therefore, the ability to accurately predict what your customers will do next is limited, out of date, at best.
- Traditional models are built on a predefined set of datasets, typically limited to demographic aggregates, which inhibits the ability to know more about what your customers are doing, where, when and, most importantly, why.
- Traditional RFM models have to be manually adjusted to account for shifts in markets or customers, or to focus on specific demographics and markets. This consumes precious time that should be used elsewhere to generate the results you need to hit.
- Traditional RFM models don’t provide contextual information related to customers, such as the impact of a pandemic, a recession or a socio-political conflict. Today, it’s important to have this ever-changing information in which businesses operate and which should be considered in understanding, segmenting and engaging customers.
To address the shortcomings of traditional RFM models, we have developed something special which I believe is the future of how retail marketers leverage integrated technologies to make better informed decisions.
Our pre-built, ready-to-use RFM model leverages the full capabilities of our Hyper-Integration Platform, Xfuze, to provide you with greater insights to help you:
- Identify new sales opportunities with your customers
- Understand which acquisition campaigns are the most profitable
- Identify patterns in the reasons for customers to return items to build strategies to avoid them to continue occurring
- Highlight which discount strategies work best: vouchers/coupons, bundles, so more advanced markdown strategies could be developed
- And much more….
The new level of business value generated by our RFM model is made possible by leveraging AI-driven segmentation that automatically spots patterns of shared behaviour within your customer base. This shines light on your customers, helping you to identify which of your customers hold the most value.
What does our RFM model look like in practice? Here’s a screenshot of one of our dashboard tabs.
This interactive dashboard estimates the likelihood of each of your customers to purchase again in the next 12 months. Packing more AI power and data than traditional RFM models, our smart tool utilises three different data science models that operate in conjunction with one another to identify the likelihood of return and potential spend of key customer cohorts so you can target them for retention. Our RFM model also incorporates projection data, sales data and advertising data to provide two alternative views of projected sales for the next four quarters as a default but it can look further into the future, or more granularly such as monthly.
Future of Retail Insights
Over the last few years, retailers have made huge strides in modernising their capabilities to understand, predict and engage customers. There is still more work to be done, but the pace of innovation is accelerating as marketing teams, in particular, embrace new technologies that maximise the full potential value of their organisation’s data to effectively win, grow and retain customers.
To learn more about our retail insights capabilities and our accelerator for retailers, check out our website.
Author
Lance Mercereau
Chief Marketing Officer
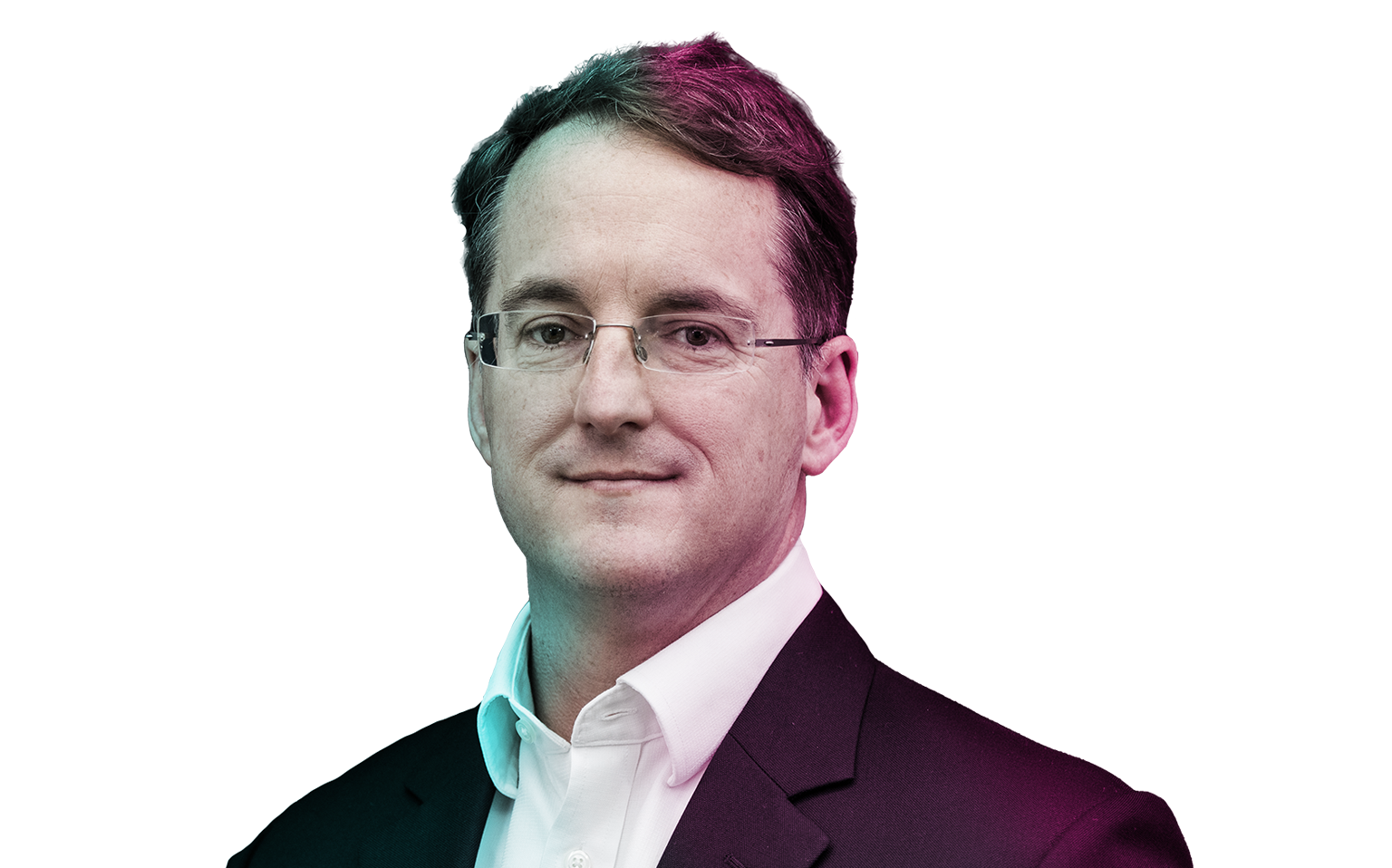
Subscribe to The Hyper-Insights Newsletter
Discover the benefits of a Hyper-connected business with infinite possibilities. Bringing the latest news and tips to your inbox from our expert team.